Method
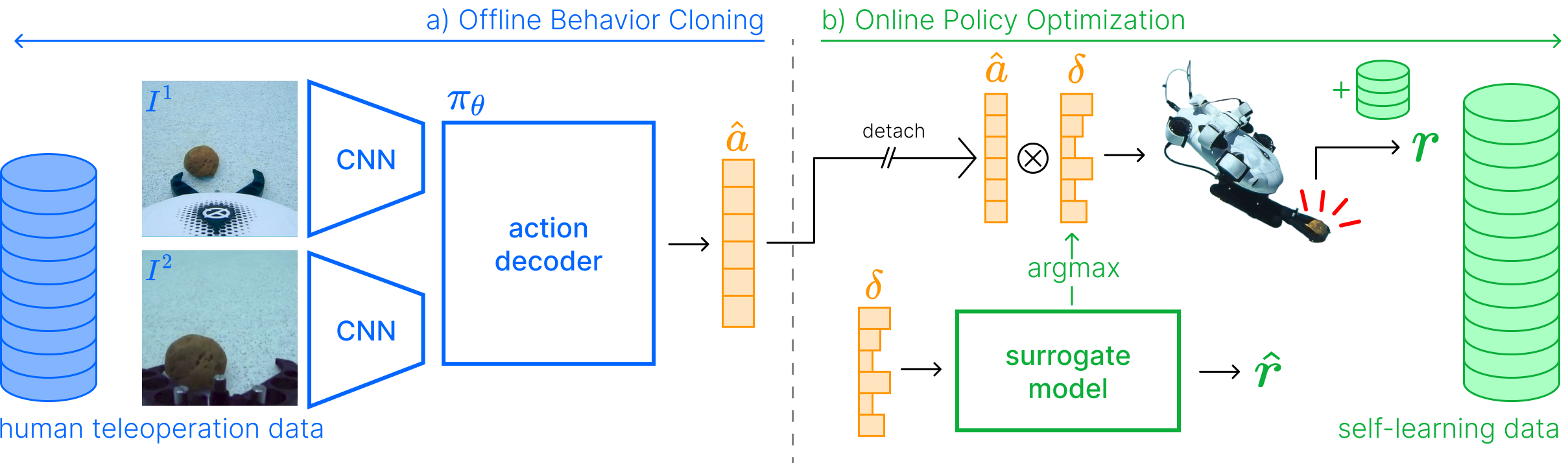
Underwater robotic manipulation faces significant challenges due to complex fluid dynamics and unstructured environments, causing most manipulation systems to rely heavily on human teleoperation. In this paper, we introduce AquaBot, a fully autonomous manipulation system that combines be- havior cloning from human demonstrations with self-learning optimization to improve beyond human teleoperation performance. With extensive real-world experiments, we demonstrate AquaBot’s versatility across diverse manipulation tasks, including object grasping, trash sorting, and rescue retrieval. Our real-world experiments show that AquaBot’s self-optimized policy outperforms a human operator by 41% in speed. AquaBot represents a promising step towards autonomous and self-improving underwater manipulation systems. We open-source both hardware and software implementation details.
We would like to thank Cheng Chi, Aurora Qian, Yunzhu Li, Zeyi Liu, Matei Ciocarlie, and Xia Zhou for their helpful feedback. We would also like to acknowledge the technical support from QYSEA. This work is supported in part by NSF Award #2143601, #2037101, and #2132519, #1925157, Sloan Fellowship. The views and conclusions contained herein are those of the authors and should not be interpreted as necessarily representing the official policies, either expressed or implied, of the sponsors
@misc{liu2024selfimprovingautonomousunderwatermanipulation,
title={Self-Improving Autonomous Underwater Manipulation},
author={Ruoshi Liu and Huy Ha and Mengxue Hou and Shuran Song and Carl Vondrick},
year={2024},
eprint={2410.18969},
archivePrefix={arXiv},
primaryClass={cs.RO},
url={https://arxiv.org/abs/2410.18969},
}